AI-enabled Rapid Intelligent Systems Engineering
We report on an exploratory project that leverages Artificial Intelligence (AI), specifically Foundation Models and Generative AI, to enhance the quality and speed of Systems Engineering (SE) practice. This project addresses the significant issue of cost overruns and delays, as evidenced by multiple Programs of Record in the DoD. Our aim is to demonstrate the feasibility of AI augmentation in achieving revolutionary efficiency gains (i.e., speed, cost) in the design, development, assurance, and certification of mission-critical systems, compared to state-of-the-art approaches.
Our presentation revolves around four main topics:
- Systems Engineering Socio-technical Model (SESM): We are developing a model that organizes activities, tasks, roles, and associated competencies (knowledge, skills) applied in Systems Engineering today. This model’s purpose is to identify the best opportunities for applying AI and uncover the potential benefit mechanisms that underpin the targeted efficiency gains.
- Task Complexity Model (TCM): We are also developing a characterization of system engineering problem “hardness” that elucidates the main dimensions qualifying and quantifying system design, decomposition, or formalization challenges. The TCM forms the basis for measuring the scalability of AI-enabled systems engineering approaches. In other words, it answers the question of what it means for a problem to be “hard” or “big.”
- Foundation Model Technology Stack (FMTS): This technology stack aids in understanding all alternatives for applying, fine-tuning, and integrating Foundation Model technologies with other tools, including other AI-based tools. These FMTS configurations aim to remove the limitations associated with consumer-oriented AI capabilities, e.g., so-called hallucinations in LLMs, and boost capabilities relevant to high assurance system design. We have a particular interest in formal reasoning and simulation tools that help ground the AI’s outputs, maximizing their usefulness and validity.
- Evaluation Framework: We are developing a series of structured experiments that exercise aspects of the SESM. These experiments are applied to use cases characterized by a specific complexity (SDC), where we can compare baseline data obtained by measuring the effectiveness of state-of-practice approaches, e.g., manual, against the human-AI team. Here, AI refers to a specific configuration of the Foundation Model Technology Stack.
The outcomes of these efforts aim to guide decision-makers who wish to make evidence-backed investments in AI technologies, considering their current state in terms of their affordances and weaknesses.
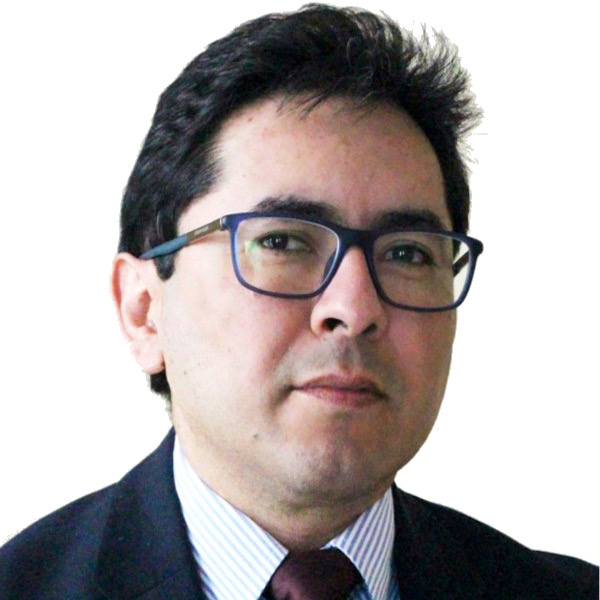
Dr. Mauricio Castillo-Effen is a Fellow at Lockheed Martin Advanced Technology Laboratories (LM ATL), where he leads the research area in Trustworthy AI and Autonomy (TAA). His team focuses on developing solutions for deploying complex decision making in high criticality applications, collaborating closely with Lockheed Martin’s Business Areas to address challenges related to Verification, Validation, Test, Evaluation, and Certification.
He has served as Principal Investigator and contributor for multiple R&D programs funded by DARPA, AFRL, NASA, and DHS advancing the fields of autonomy, assurance, and certification in the aerospace industry. He has a background in systems theory, control and estimation, cyber physical systems, and robotics.
He has also taught at multiple universities worldwide. He holds more than twenty patents in robotics, autonomy, and aviation. Dr. Castillo-Effen received his Ph.D. in Electrical Engineering from the University of South Florida.